
INDEXED BY
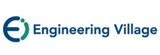 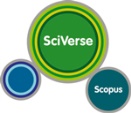

CONFERENCE TO BE HELD IN
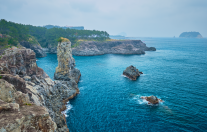
Jeju Island, South Korea

SPONSORED BY
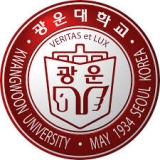
SUPPORTED BY
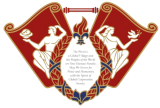
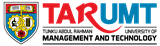
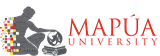
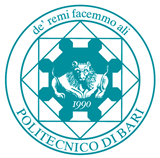
|
KEYNOTE
SPEAKERS of ICBET 2024

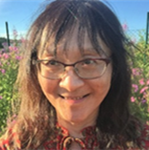
Prof. Irene Yu-Hua Gu
Chalmers University of Technology, Sweden
Dr. Irene Yu-Hua Gu received Ph.D. degree
in electrical engineering from Eindhoven University of
Technology, Eindhoven, The Netherlands, in 1992. From 1992
to 1996, she was Research Fellow at Philips Research
Institute IPO, Eindhoven, The Netherlands, post dr. at
Staffordshire University, Staffordshire, U.K., and Lecturer
at the University of Birmingham, Birmingham, U.K. Since
1996, she has been with the Department of Electrical
Engineering (previous name: Department of Signals and
Systems), Chalmers University of Technology, Gothenburg,
Sweden, where she has been a Professor since 2004. Her
research interests include statistical image and video
processing, video object tracking and recognition, machine
learning and deep learning, and signal processing with
applications. During the last several years her main
research has been focused on biomedical image analysis and
deep learning. Dr. Gu was an Associate Editor for IEEE
Transactions on Systems, Man, and Cybernetics, Part A:
Systems and Humans, and Part B: Cybernetics from 2000 to
2005, and Associate Editor for EURASIP Journal on Advances
in Signal Processing from 2005 to 2016, Editorial Board of
the Journal of Ambient Intelligence and Smart Environments
from 2011 to 2019. She is a Senior Area Editor for IEEE
Signal Processing Letters since 2021. She was elected as the
Chair of the IEEE Swedish Signal Processing Chapter from
2001 to 2004. She is a senior member of IEEE. She has
coauthored over 200 papers, and has been ranked as the top
50 scientists in the field of Computer Science and
Electronics in Sweden in the 6th Edition of 2020 ranking by
Guide2Research team.
Speech Title: "Federated Deep
Learning and its Application to Brain Cancer Molecular
Subtype Prediction from MRIs"
Abstract: One of the most common types of
brain cancer is glioma. Identification of glioma subtypes is
essential for clinicians to decide treatment strategies. If
MRI brain scans show tumors, biopsies are usually followed.
Previous studies show that deep learning-based classifiers
are promising for predicting glioma subtypes from MRI scans
of new patients without using biopsies. These classifiers
are formed by deep networks trained by MRI datasets with
tumor subtypes as annotations. Many challenges remain for
such classifiers on achieving high performance useful to
clinical applications. Apart from requiring large MRI
training datasets or combining multiple small MRI datasets,
data protection and privacy from different
hospitals/regions/countries pose heavy constrains on sharing
training datasets. Federated learning offers the possibility
that each hospital can hold its own training dataset without
sharing, while still obtain a local classifier with nearly
the same performance as that of a centrally trained
classifier by using all datasets. In this talk, we first
review several federated deep learning approaches, followed
by dedicated federated learning for glioma classification
especially for glioma subtypes. We show results that
federated learned classifiers may achieve almost similar
performance as that of centrally learned classifiers. This
is encouraging for further AI/DL research towards clinical
applications.
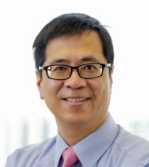
Prof. Stephen Kwok-Wing Tsui (h-index: 58)
The Chinese University of Hong Kong, Hong Kong
Stephen Kwok-Wing Tsui is currently a
Professor and the Associate Director (Research) in the
School of Biomedical Sciences. He is also the Director of
Hong Kong Bioinformatics Centre in the Chinese University of
Hong Kong (CUHK). In 1995, he received his PhD degree in
Biochemistry at CUHK. He was then appointed as an Assistant
Professor in the Biochemistry Department in 1997 and
promoted to the professorship in 2004. He was also a former
member of the International HapMap Consortium and worked on
the single nucleotide polymorphisms of human chromosome 3p.
During the SARS outbreak in 2003, his team was one of the
earliest teams that cracked the complete genome of the
SARS-coronavirus and facilitated the emergence of real-time
PCR assay for the virus. Totally, he has published more than
240 scientific papers in international journals, including
Nature, Nature Machine Intelligence, New England Journal
of Medicine, Lancet, PNAS, Nucleic Acids Research, Genome
Biology and Bioinformatics. His h-index is 58 and the
citations of his publications are over 20,000. His major
research interests are next generation sequencing,
bioinformatics and metagenomics in human diseases.
Speech Title: "Genomics Study Reveals
Insights into the Divergent Evolution and Comprehensive
Allergen Profiles of Astigmatic Mites"
Abstract: Highly diversified astigmatic
mites comprise many medically important human household
pests such as house dust mites causing ~ 1-2% of all
allergic diseases globally. However, their evolutionary
origin and diverse lifestyles have not been illustrated at
the genomic level, which hampers allergy prevention and our
exploration of these household pests. Using six high-quality
assembled and annotated genomes, this study thoroughly
explored the divergence of Acariformes and the
diversification of astigmatic mites. Within astigmatic
mites, a wide range of gene families rapidly expanded via
tandem gene duplications. Gene diversification after tandem
duplications provides many genetic resources for adaptation
to sensing environmental signals, digestion, and
detoxification in rapidly changing household environments.
Throughout the evolution of Acariformes, massive horizontal
gene transfer events occurred in gene families enable
detoxification and digestive functions and provide perfect
drug targets for pest control. This genomics study sheds
light on the divergent evolution and quick adaptation to
human household environments of astigmatic mites and
provides insights into the genetic adaptations and even
control of human household pests. Moreover, in this talk an
innovative and efficient way to unveil the comprehensive
allergen profiles of astigmatic mites will be described.
INVITED
SPEAKERS

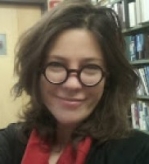
Prof. Dilek Çökeliler Serdaroğlu
Başkent University, Turkey
Dilek Çökeliler Serdaroğlu is a professor
and chair in the Department of Biomedical Engineering at
Baskent University, Ankara, Turkey (Accredited by MUDEK).
Dr. Çökeliler received his Ph.D. from Hacettepe University,
Ankara, Turkey including one year international doctoral
training experience study entitled “plasma aided
immunosensor fabrication” in Charles University,
Macromolecular Physics Department in Czech Republic.
Moreover, she won “Post Gradual Study Support” Funded by The
Ministry of Education of Czech Republic, at the Department
of Physics, J.E. Purkyne University. She was post doctoral
researcher in the Biological Systems Engineering Department,
University of Wisconsin Madison,USA. Now, her research and
teaching activities at Başkent University have emphasized on
both various aspects of plasma surface modifications of
biomaterials and nanofiber production with electrospinning
technique / nanomaterial-based mass sensitive sensors. She
is the recipient of several international patent awards
(number: +8) including the L’ORÉAL Awards for Women in
Science in 2009 In addition, she is a regular lecturer in
the Intensive Summer Course: Biomedical Engineering in an
International Perspective in Jade Hochschule Wilhelmshaven,
Applied Engineering Science Department, Germany for 15
years.
Speech Title: "Glow Discharge Plasma
Technology for Biomedical Applications and Integration
Design of Experiment Model for In-Vitro and In-Vivo Test"
Abstract: This presentation evaluates the
performance of a focused plasma-based surgical prototype
device for use in spine discectomy surgery, including
identifying active device operating conditions through
engineering models and in-vitro tests. It also discusses
experiences related to antibacterial efficacy, creating an
appropriate in-vivo wound model, and evaluating the healing
process to demonstrate the effect of plasma irradiation on
anastomosis. Plasma is a partially ionized gas mixture with
highly reactive ions and electrons, neutral atoms, electric
fields, reactive molecules, induced species, and UV
radiation resulting from exposing a substance to solid
energy in the gas direct plasma discharge application to
living cells, bacteria, or tissues, studied for its
potential in disinfection, healing, and cancer treatment.
The design of the experiment analysis is crucial in
engineering, improving production processes and
optimization, and revealing changes in output due to input
variable changes. Experimental design methods are used to
understand factors like plasma charge neutralization,
observe optimal factor combinations, show statistical
significance, and determine successful factor levels. This
presentation includes in-vitro and in-vivo experiences,
using a complete factorial experimental design in
plasma-based device development.
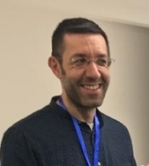
Assoc. Prof. Giovanni Pappalettera
Polytechnic University of Bari, Italy
Giovanni Pappalettera is an Associate
Professor at Polytechnic University of Bari, where he also
lectures in the Bachelor Degree in Industrial Design and the
Master Degree of Mechanical Engineering. He earned his PhD
in Mechanical and Biomechanical Design at Politecnico di
Bari and in Biomechanics and Bioengineering at Scuola
Interpolitecnica di Dottorato di Torino. His primary
research interests include material characterization and
experimental mechanics, encompassing optical methods,
acoustic emission, and residual stress analysis. He is
actively involved in biomechanics research including
ultrasound-induced fatigue effects on cells, mechanical
characterization of dental devices, and 3D dental element
reconstruction. In 2007 and 2008, he was a visiting
researcher at Politechnika Warsawska – Warszawa, and in
2009, he was a visiting researcher at the Northern Illinois
University - DeKalb. Giovanni serves on the PhD committee in
Mechanical and Management Engineering at Politecnico di
Bari. He has authored over a hundred papers in international
journals and conference proceedings and is a co-inventor of
three Italian patents. He is also a co-author of three books
and a member of international associations, including the
Society of Experimental Mechanics, the European Society of
Experimental Mechanics, and the Italian Association of
Stress Analysis. Since 2003, he has been a research
associate at the National Institute of Nuclear Physics
(INFN).
Speech Title: "Effects of OUT
(Onco-Ultrasound-Tripsy) in-vitro Treatment on Cancer and
Healthy Cells: Application to U937 Human Histiocytic
Lymphoma Cells"
Abstract: In recent years, research has
made many progresses in understanding and treating cancer.
Different types of tumours can now be treated successfully,
allowing a long-life expectancy after the disease. However,
the interest towards alternative clinical approaches is
alive and one of the current challenges consists in
identifying treatments able to act selectively on tumour,
without harming the surrounding environment. Several studies
have highlighted the ability of low-intensity ultrasound to
selectively damage tumour cells, based on the different
mechanical properties that they present compared to healthy
cells. In this study, we analyzed the ability of ultrasound
to damage U937 Human Histiocytic Lymphoma cells, without
altering the viability of the corresponding healthy Human
CD14+ Monocytes cells, used as control. Different sonication
parameters were tested, to identify the conditions that
allow the best results in terms of selective death of U937
cancer cells to be achieved. Informativa Privacy - Ai sensi
del Regolamento (UE) 2016/679 si precisa che le informazioni
contenute in questo messaggio sono riservate e ad uso
esclusivo del destinatario. Qualora il messaggio in parola
Le fosse pervenuto per errore, La preghiamo di eliminarlo
senza copiarlo e di non inoltrarlo a terzi, dandocene
gentilmente comunicazione. Grazie. Privacy Information -
This message, for the Regulation (UE) 2016/679, may contain
confidential and/or privileged information. If you are not
the addressee or authorized to receive this for the
addressee, you must not use, copy, disclose or take any
action based on this message or any information herein. If
you have received this message in error, please advise the
sender immediately by reply e-mail and delete this message.
Thank you for your cooperation.
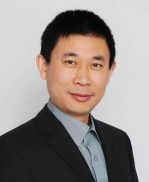
Assoc. Prof. Guanghui (Richard) Wang
Toronto Metropolitan University, Canada
Guanghui (Richard) Wang received the
Ph.D. degree in engineering from the University of Waterloo,
Waterloo, ON, Canada, in 2014. He is currently an Associate
Professor with the Department of Computer Science, Toronto
Metropolitan University (TMU), Toronto, ON. He is also the
director of the Computer Vision and Intelligent Systems
Laboratory at TMU. From 2014 to 2020, he was an Assistant
Professor and then an Associate Professor in the Department
of Electrical Engineering and Computer Science at the
University of Kansas (KU), Lawrence, KS, USA. He has
authored one book “Guide to Three Dimensional Structure and
Motion Factorization”, published by Springer-Verlag. He has
authored or co-authored more than 180 papers in
peer-reviewed journals and conferences. His research
interests include computer vision, image analysis, machine
learning, and intelligent systems.
Speech Title: "Polyp Segmentation
from Colonoscopy Images Using Enhanced Neural Networks"
Abstract: Colonoscopy is a crucial
procedure for detecting colorectal polyps, which stand as
the primary precursors to colorectal cancer. However,
accurately segmenting polyps presents a significant
challenge due to their diverse shapes, sizes, colors, and
texture variations. To address these challenges and enhance
polyp segmentation performance, we propose two enhanced
neural network structures. The first network enhances
semantic information through a novel Semantic Feature
Enhancement Module (SFEM) and an Adaptive Global Context
Module (AGCM). By integrating these modules, we
progressively refine feature quality across layers, thereby
enhancing the final feature representation. The second
network introduces a novel Fuzzy Attention module, designed
to prioritize difficult pixels, particularly those near the
boundaries that pose a greater challenge for prediction.
This attention module can be seamlessly incorporated into
any backbone network. We evaluated its efficacy with three
backbone networks: Res2Net, ConvNext, and Pyramid Vision
Transformer. Our proposed approaches are rigorously
evaluated across five colonoscopy datasets, showcasing
superior performance compared to other state-of-the-art
models.
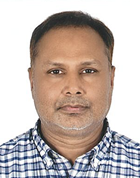
Assoc. Prof. Md. Altaf-Ul-Amin
NARA Institute of Science and Technology, Japan
Md. Altaf-Ul-Amin received B.Sc. degree
in Electrical and Electronic Engineering from Bangladesh
University of Engineering and Technology (BUET), Dhaka,
M.Sc. degree in Electrical, Electronic and Systems
Engineering from Universiti Kebangsaan Malaysia (UKM) and
PhD degree from Nara Institute of Science and Technology
(NAIST), Japan. He received the best student paper award in
the IEEE 10th Asian Test Symposium. Also, he received two
other best paper awards as a co-author of journal articles.
He previously worked in several universities in Bangladesh,
Malaysia and Japan. Currently he is working as an associate
professor in Computational Systems Biology Lab of NAIST. He
is conducting research on Network Biology, Systems Biology,
Cheminformatics and Biological Databases. He published
around 90 peer reviewed papers in international journals and
conference proceedings. Current google scholar citation
index of his publications is more than 7900.
Speech Title: "Computational Approaches to
Predict Natural Antibiotics based on Traditional Herbal
Medicines"
Abstract: Antibiotic resistance is a
major public health threat and there is an urgent need for
new antibiotics. Traditional herbal medicine systems, such
as Jamu, Unani, and Traditional Chinese Medicine, have been
used for centuries to treat bacterial infections. Machine
learning methods have been shown to be effective for
predicting potential natural antibiotic candidates based on
traditional herbal medicine systems. In this study, we used
machine learning methods to predict potential natural
antibiotic candidates at plant and metabolite levels. We
evaluated different machine learning algorithms and
preprocessing techniques to obtain the best prediction
accuracy. For Jamu, we achieved an accuracy of 91.10% using
the Random Forest model. For Unani, we achieved an accuracy
of 83% using a multilayer perceptron model with SMOTE
preprocessing. In total, we predicted 42 potential plant
candidates and 201 candidate metabolites as potential
natural antibiotics. Many of these candidates have been
validated based on published literature mentioning their
antibacterial properties. Some others are structurally
similar to known antibiotics. Our findings suggest that
machine learning methods can be used to effectively predict
potential natural antibiotic candidates utilizing
traditional herbal medicines. This approach has the
potential to accelerate the development of new antibiotics
to combat antibiotic-resistant pathogens.
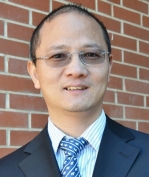
Prof. Wenqiao (Wayne) Yuan
North Carolina State University, USA
Dr. Wenqiao (Wayne) Yuan is currently a
professor at the Department of Biological and Agricultural
Engineering, North Carolina State University (NCSU),
Raleigh, NC, USA. He received a Ph.D. degree in Biological
and Agricultural Engineering from the University of Illinois
at Urbana-Champaign, Urbana, IL, USA. He has been the chair
and vice chair of several technical committees of American
Society of Agricultural and Biological Engineers (ASABE),
and is an associate editor of Journal of the ASABE,
International Journal of Agricultural and Biological
Engineering, and Applied Engineering in Agriculture. Dr.
Yuan’s research is mainly focused on bioenergy and
bioproducts, such as microalgae culture and bioprocessing,
green agriculture development, biomass thermochemical and
biochemical conversion, and microbial ad bio-fuel cells. Dr.
Yuan has published more than 200 papers and 4 book chapters,
with numerous invited speeches worldwide. He received the
“CAREER Award” in 2010 from US National Science Foundation,
the “New Holland Young Researcher Award” in 2012, the
“Engineering Concept of the Year Award” in 2016 from ASABE,
and the “Research Fellowship for Experienced Researchers”
from Alexander von Humboldt Foundation (Germany) in 2017. He
has also received nearly a dozen other prestigious
international and national awards and honor.
Speech Title: "Biochar and its
Potential Biomedical Applications"
Abstract: Biochar is a carbon-rich material resulted from the thermal conversion of biomass. It can improve environmental quality when used for pollutant removal in liquid or gas media, soil conditioning, or as a long-term storage of carbon. It has been found to have at least 50 different uses. In this presentation, a non-traditional use of biochar in biomedical engineering is discussed. Mesoporous carbon particles (MCP) were generated from activated biochar. Then Glucose oxidase (GOx) and catalase (CAT) were self-assembled on the MCPs to form a GOx/CAT/MCP composite, which was then dispersed in Nafion solution and layered on carbon cloth to fabricate a flexible 3-D bio-anode possessing extremely high electron transfer rate. Such bio-anode designed via the simple, bio-compatible, and multi-enzyme self-assembly approach displayed exceptional electrochemical performance for the fabrication of glucose-based bio-fuel cells or biosensors, which has high potential to be a self-sustained bio-battery for implantable biomedical devices or for human health monitoring, especially for diabetic patients. |